New NFI and University of Amsterdam methods for recognizing deepfakes
Deepfakes, manipulated videos, can no longer be ignored. From fake applications, people who enter into discussion with companies online to fish for commercial information, to porn videos where the head of a Dutch celebrity has been pasted on. The misuse of deepfakes is constantly increasing. The Netherlands Forensic Institute (NFI) and the University of Amsterdam have been researching this worrying phenomenon for a number of years now. ‘We now have a good combination of new methods to help us detect deepfakes.’
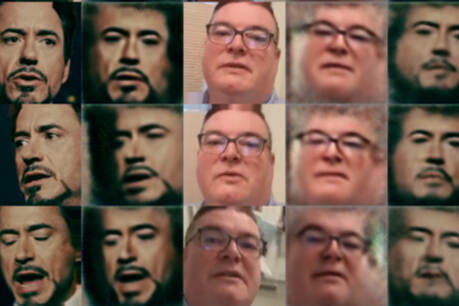
The NFI has already handled a number of cases in which deepfakes were investigated. These involved videos where the bodies and faces of individuals had been manipulated. This trend is expected to accelerate in the future. ‘Worrying’, said Zeno Geradts, forensic investigator at the NFI and professor by special appointment at the University of Amsterdam. Europol and the FBI are also warning about the misuse of deepfakes. There are even websites where you can paste the faces of celebrities onto porn videos, as if it were the most natural thing in the world. There are also examples of fraud, such as a deepfake of a manager tasking an employee with transferring a substantial sum of money.
New methods
Over the last three years, experts at the NFI have become more proficient at detecting deepfakes. To do this, they use a checklist of manual comparisons. Among other things, they look for inconsistencies in focus, sometimes the teeth are blurred but the lips are in focus. Or they check characteristics like birthmarks: are they always in the same place? But now they have also added a few new methods to their toolbox. ‘It is precisely this combination of tools that assists in the recognition of deepfakes, which are getting better all the time’, said Geradts. ‘You have to look at the total picture. So the video and the audio, the speech component. Synthetic voices are, after all, very difficult to make realistic.’
One of the new methods for analysing deepfakes is the ‘rolling shutter method’, in other words using the electrical network frequency to discover the time and location of the video. We could already get this from audio files, but getting it from video too is new. ‘The electricity from a plug socket that people have at home has a frequency of 50 hertz. This is not constant and varies between approximately 49.5 and 50.5’, explains Geradts. ‘This is the same throughout the whole of Europe. The electricity networks are, after all, connected to each other. The pattern from this generates a type of timeline: we can use it to determine when a video was recorded.’
Gentle flickering
You can see that something is not right with the recording from the light on the background of the video. For example, that the video was recorded at a time other than that claimed. Just how does that work? The camera in your phone is divided into pixels, individual sensors. The investigators can now successfully retrieve the 50 hertz signal that is generated when someone has a light on in the room for example. ‘We couldn’t do this at first,’ the investigator admits. ‘If you have a lamp on in the background, you can see the 50 hertz signal as a gentle flickering in the video. We can use this to discover when it was recorded, at night or during the day for instance. Or that the video was recorded a month ago while it is claimed to have been recorded yesterday.’ The NFI and the University of Amsterdam have published a scientific article about this method. In theory, the investigators can also determine whether a video was recorded in Paris or in Amsterdam, but they have yet to validate that method.
There’s also one other method: Photo Response Non Uniformity (PRNU), which is actually a kind of fingerprint of the camera in your phone. It can be used to determine which camera recorded the images. Geradts explains what a PRNU pattern is. ‘If you shine an equal amount of light on a specific camera sensor, each individual pixel reacts a little bit differently. This provides opportunities for deepfake detection. After all, the pixels produce a specific pattern. We can then compare that pattern with the pattern of a deepfake: does it match the ‘fingerprint’ of a specific camera? This allows us to determine which device the images come from. If we know the camera the images are claimed to come from, we can now successfully investigate that’, said Geradts.
Combination of methods
The power of good deepfake detection lies in the use of a combination of classical methods and artificial intelligence. There is now also a new method for the audio component of deepfakes. The scientific publication on this is almost complete. If someone has imitated a speaker, there is now a tool to detect this. Sometimes this can simply be heard, but you can now make it visible. ‘The use of algorithms for explainable AI now allows us to visualise whether the audio has been manipulated, and is therefore a deepfake voice’, according to Geradts. If the voice has not been manipulated, we see a coloured surface. If manipulation has occurred, the surface has a distinct pattern. This will have to be evaluated by an expert before it can be used as (supplementary) evidence.
Collaboration
Although deepfakes are becoming even better and more realistic, there are good tools for the successful detection of deepfakes. ‘We will have to keep on doing research and discovering new methods to stay ahead of the criminals’, said Geradts. ‘It continues to be a cat and mouse game.’